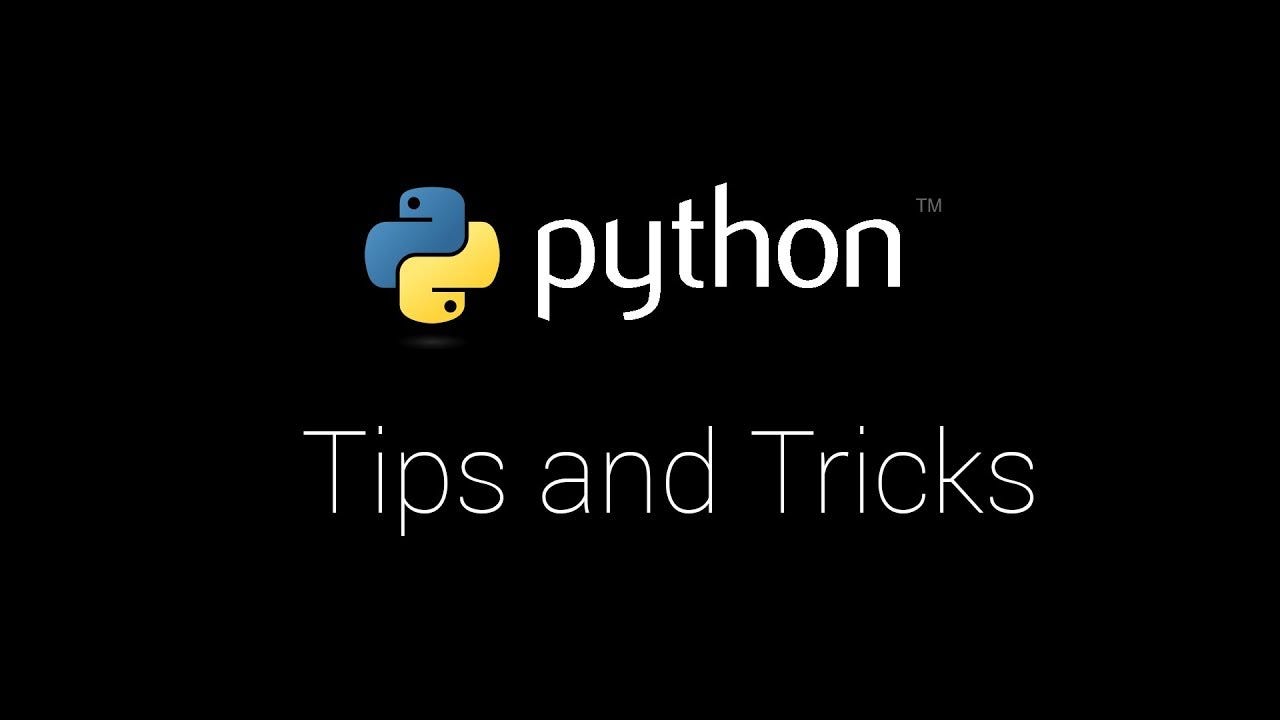
Python list methods
append(item)
Python string methods
isalpha()
append(item)
>>> lst = [1, 2, 2]count(item)
>>> lst.append(4)
[1, 2, 2, 4]
>>> lst.count(2)extend(list)
2
>>> lst.extend([5, 6])index(item)
[1, 2, 2, 4, 5, 6]
>>> lst.index(5)insert(position, item)
4
>>>lst.insert(1, 9)sort()
[1, 9, 2, 2, 3, 5, 6]
>>> lst.sort()pop(index)
[1, 2, 2, 3, 5, 6, 9]
>>> lst.pop()reverse()
9
>>> lst.reverse()
[6, 5, 3, 2, 1, 1]
Python string methods
>>> s = 'Hello2Python'count(sub, start, end)
>>> s.count('l', 1, 5)find(sub, start, end)
2
>>> s.find('l', 0, 5)index(sub, start, end)
2
>>> s.index('t', 0, 9)isalnum()
8
>>> s.isalnum()
True
isalpha()
>>> s.isalpha()isdigit()
False
>>> s.isdigit()islower()
False
>>> s.islower()isupper()
False
>>> s.isupper()isspace()
False
>>> s.isspace()join()
False
>>> s.join('___')lower()
'_Hello2Python_Hello2Python_Hello2Python_'
>>> s.lower()partition(sep)
hello2python
>>> s.partition('2')split(sep)
('Hello', '2', 'Python')
>>> s.split('2')strip()
['Hello', 'Python']
>>> s.strip('n')upper()
'Hello2Pytho'
>>> s.upper()
'HELLO2PYTHON'